
Recency of your product feedback matters
AI in 2025: Overcoming Challenges from PoC to Production
Artificial intelligence (AI) is no longer just a promising technology. Even in 2025, this will be a key tool for companies to gain an advantage. From creating a PoC (proof of concept) to actual production, there are many challenges. It is about detailed scaling, maintaining security compliance and data quality, and more.
This is a challenging way for platforms with advanced machine learning (ML) algorithms and huge amounts of detailed customer data. It is important to note that the most critical success factors will be tuning the models, integrating existing business processes, and stable performance.
In this comprehensive guide, we will highlight the main obstacles on the path from PoC to AI implementation in business and consider the effective ways of overcoming them.
Hype vs reality: the truth about AI's impact
As AI technology increases, the hype often distorts views of capability and limitation. In this section, we review where the dividing line is set between inflated expectations and real gains that can be achieved using AI.
AI hype: unrealistic expectations
Artificial intelligence has become very popular in the last few years, generating many expectations about its capabilities. Media, advertising, and even tech giants create a semblance that AI can solve any task, automate business processes without human interference, and is way more productive than human intelligence in making decisions.
But in reality, it is much more complicated. With the progress of machine learning, natural language processing (NLP), natural language generation (NLG), and generative AI, most companies still face obstacles that cannot easily be implemented into real business practice.
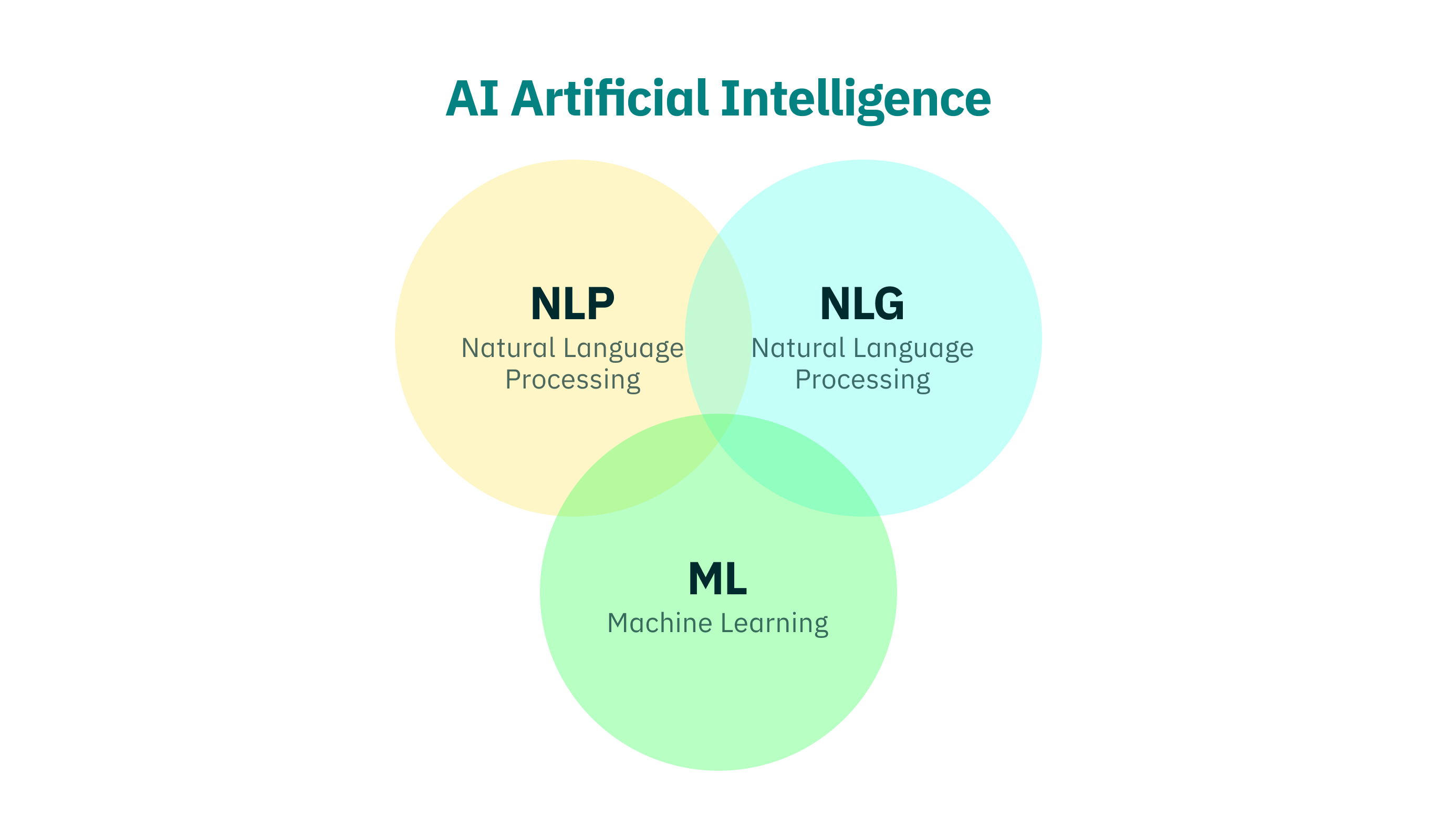
In particular, you can hear the following statements:
AI will replace people in all professions
In fact, AI is a helpful tool, but it can't operate alone. While companies claim AI has reasoning skills, it still needs humans for accurate decision-making and problem-solving. It can automate tasks like data processing and basic customer service, but people are needed for more complex issues. Also, AI support now goes beyond chat, with voice AI startups growing.
ML provides completely accurate predictions
Even the best AI models are only 80-90% accurate, and reaching that level takes a lot of work and effort. However, this still leaves room for errors. Particularly, this critical issue may touch such spheres as finance, medicine, or even legal affairs because minor deviations will result in terrible consequences.
AI works autonomously without the need for human intervention
In real life, any model needs to be constantly monitored, adapted to changes in data, and updated with algorithms. If not monitored properly, even the most advanced models may produce wrong results due to data shifts or new, unpredictable scenarios.
AI is fast and cheap to implement
AI solution deployment is a complex process. This involves significant investments in infrastructure, computing resources, data collection and labeling, and training staff. This can take several months or even years for midsize businesses.
Despite the hype, companies should take a pragmatic view of AI, considering the actual cost and efficacy of the technology within a particular business context.
The reality of implementing AI in business
However, AI implementation into a corporate environment is incomparably more complex than it may seem at first sight. Even if a company recognizes the need for AI-based automation and analytics, a number of serious challenges lie ahead that can delay its actual implementation.
Data is the main obstacle to AI effectiveness
AI models need data, but, in fact, the quality is what heavily relies on the predictions. A problem is that too many organizations face such factors:
- Incomplete or unstructured data: for example, quite often, a lot of fragmented pieces of information are provided because some data may be stored in different CRM systems, different spreadsheet types, or sometimes even in simple paper format.
- Noise in data: errors, duplicate records, and irrelevant or outdated information can seriously affect the quality of AI models.
- Lack of required data: quite often, an enterprise cannot gather an appropriate volume of quality data due to legislative restrictions, such as GDPR or CCPA, or because the data collection process is too difficult.
Testing and validating models
Many companies pass the Proof of Concept stage, yet not all move to production. That is due to the difficulty in testing how effective the model will be under real conditions.
It is possible that under laboratory conditions, the models are very efficient and simply do not work on real data. For example, a model that predicts the demand for goods could work perfectly on historical data but work much worse in the case of sudden changes in consumer behavior.
The biggest challenge is that there is no standard way of assessing the correctness of AI solutions. Every domain has its set of metrics, and it isn't always simple for businesses to determine whether the model is yielding the desired results.
The more complex the model, the harder it is to explain why it made a certain decision. This is especially important for the financial sector and medicine, where transparency of algorithms is critical.
Integration into existing business processes
Even if some AI model shows great results, its implementation in a real business process may suffer from the following difficulties:
- Limitations of the infrastructure. The effective work of many algorithms requires serious servers, cloud computing, and well-established APIs for integration with existing company systems.
- Resistance among employees. Such employees fear a loss of their jobs due to automation. This can cause internal resistance to technology implementation.
- Training the staff. While working with AI, the use of new tools, analysis, and decision-making based on model results are required.
- Security concerns. It involves implementing AI systems into business solutions with new data security risks. Companies must ensure that any AI solution harmonizes with data protection legislation, that sensitive data is encrypted, and that there is intrusion or data spillage protection.
AI won’t replace people, but it will transform jobs
You may have heard that the development of artificial intelligence has sparked much discussion about the future of human work. Some predict a scene where robots and algorithms take over completely. Others insist that AI strategy for business will be used only to complement human activity and enhance productivity.
As with everything, the truth lies somewhere in between: artificial intelligence will not take people out of work, but it will change the nature of work beyond all recognition. This change will be demanded from businesses and workers.
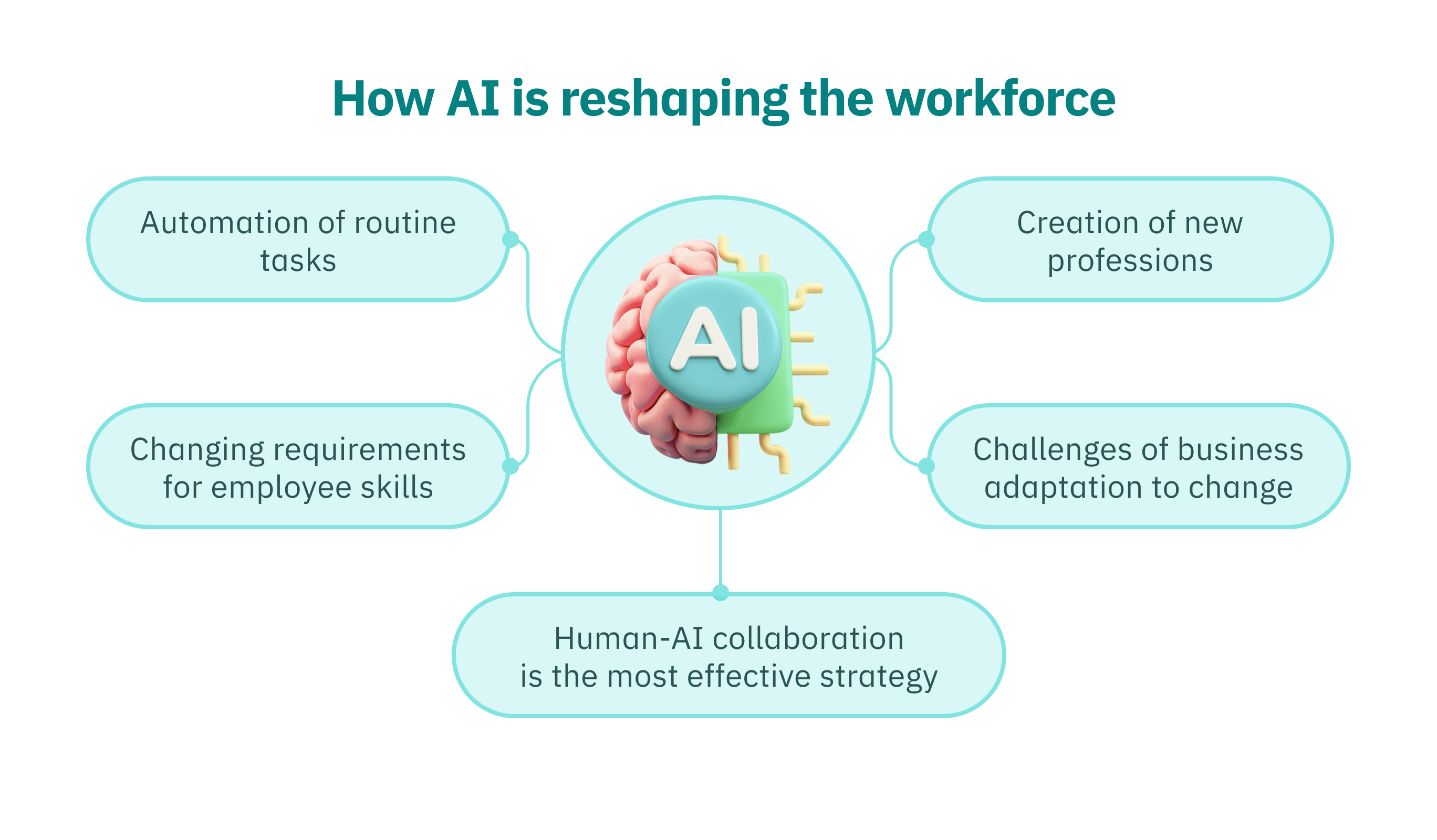
Automation of routine tasks
The most basic effect of AI on the labor market is the automation of tasks that have required a lot of time in the past. For example:
Administrative processes
Plenty of routine operations (document processing, financial management, data entry, and reporting) can be performed by means of artificial intelligence much quicker and without any mistakes.
Customer support
There are AI-run chatbots/voice assistants to respond to 24/7 user requests while automating a large number, up to 80%, in banking, ecommerce, and other industries.
Big data analytics
AI in businesses helps analyze trends and find patterns in customer behavior, which predictively and strongly enhances the effectiveness of marketing and financial strategy implementations.
On the other hand, automation does not mean the refusal of human labor in general. On the contrary, this shifts the focus of work: instead of performing routine operations, workers will concentrate on more strategic and creative aspects of their activities.
Creation of new professions
While artificial intelligence is displacing some kinds of jobs, it gives a strong impulse to the emergence of completely new occupations that have not been in demand before. These include:
- Machine learning engineers: There is a great demand for specialists who create and optimize AI algorithms.
- Operators and analysts of AI systems: For algorithms to function properly, people should be involved who can assess their performance, adjust models, and eliminate errors.
- Ethics specialists in AI: As AI implementation touches every aspect of life, there is a need for people who will check on the compliance of ethical standards and transparency in using data.
- Creative professions using AI: Designers, marketers, writers, and video editors all use AI algorithms to automate part of the processes and free time for creative tasks.
Changing requirements for employee skills
While AI is being implemented in one area after another, companies are changing the requirements for people working for them. Specifically, now there is an ever-growing demand for the following skills:
Analytical thinking: Automated systems generate a great amount of information that has to be interpreted properly and used to make decisions.
Flexibility and readiness to learn: Technological progress is very fast, and employees should be ready to update their knowledge and skills all the time.
Creativity: While AI may be good for performing analyses and forecasts, truly unconventional thinking, innovation, and creative vision remain exclusively human competence.
Emotional intelligence and communication: The human factor is still crucial in many areas, especially in customer service. Interaction with customers, negotiations, and personnel management requires a deep understanding of human behavior, which AI cannot yet fully replace.
Challenges of business adaptation to change
Companies will be obliged to change technically, and in this way, business processes and organizational culture are organized. The main difficulties arise in the following components:
Reorganizing the work processes: Automation is changing business structures, and companies have to think about the role of employees and optimize collaboration between humans and AI.
Investing in personnel training: Instead of reducing the labor force, a company is better off investing resources in personnel development so that their staff can successfully apply new technologies.
Building trust in AI: Many workers are skeptical about automation and immediately think of losing jobs. Therefore, it is very important to explain how technology will help make their jobs easier, not replace them.
Making sure AI serves ethical purposes: Companies must consider issues such as algorithmic transparency, data protection, and social responsibility while implementing new solutions.
Solve issues from PoC to production with Unwink.AI. Start transforming your AI today! Get in Touch
Human-AI collaboration is the most effective strategy
The most successful companies already understood that the best results can be achieved not by the complete replacement of people with AI but by their effective interaction. The best examples of such collaboration are:
- Medicine: Doctors use AI algorithms for faster diagnosis, but the final decision is made by a human.
- Finance: AI helps detect fraud and analyze the market, but investment decisions are left to specialists.
- Law: Algorithms process legal documents, finding what is needed, thus saving a great deal of time for lawyers.
- Retail: With personalized AI-based recommendations, sales increase, yet customers still like live service.
How to overcome AI implementation challenges
Artificial intelligence continues to change the business landscape in 2025, delivering new analysis and process automation opportunities. However, several obstacles arise for companies wanting to implement AI into their workflows, from PoC to production. Let's analyze the main problems and some efficient ways to resolve them.
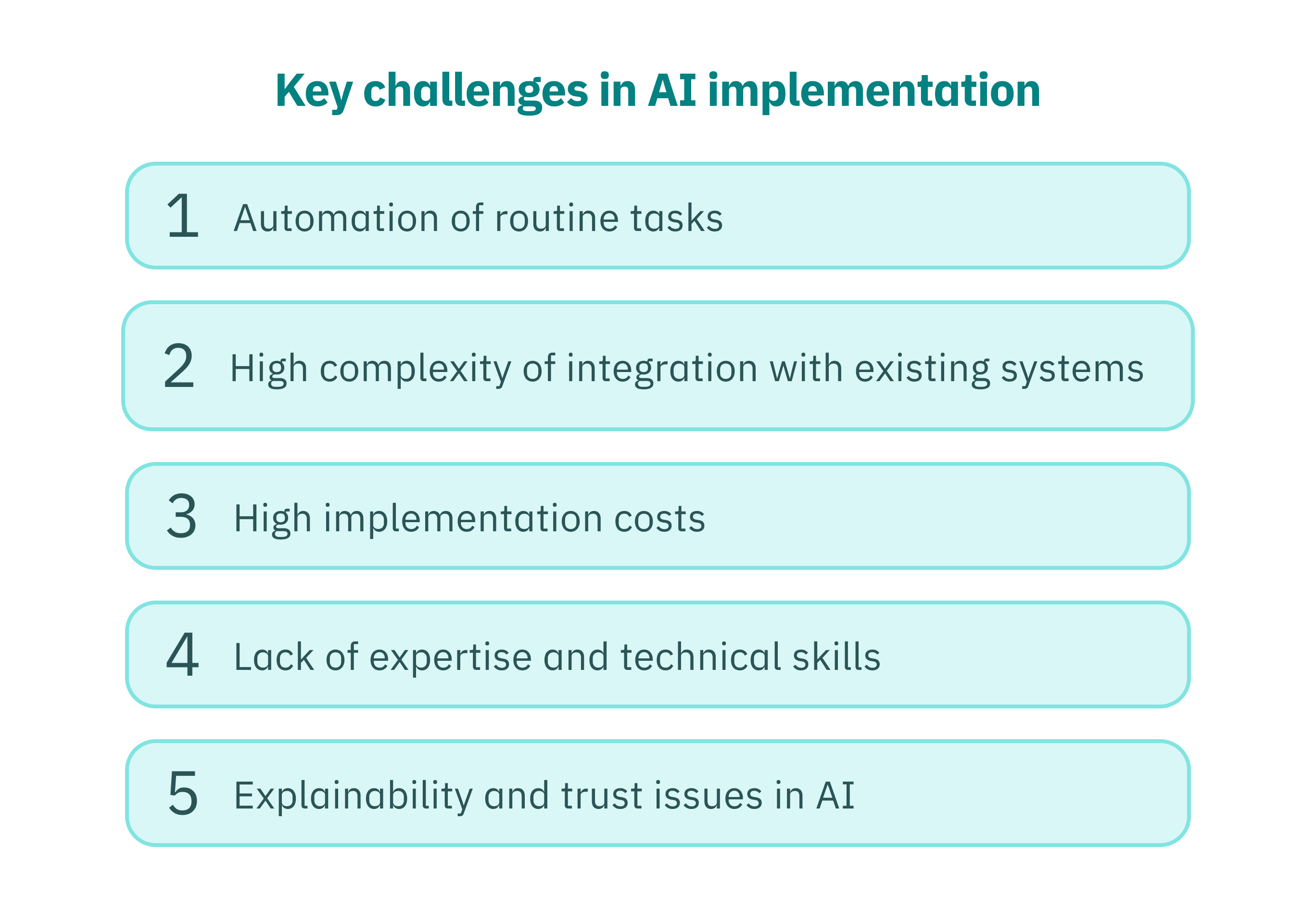
Insufficient quality and volume of data
AI algorithms require high-quality data. In cases where this information is incomplete, unrepresentative, or biased, then it forms the basis for increasingly inaccurate predictions, leading to unreliable results. Often, larger training data sets are either unavailable to the company or raise privacy issues.
Solutions
Designing the data collection and preparation AI strategy for business, finding sources, establishing the quality rules, and automating the processing will reduce manual errors. Use synthetic data generation methods to enhance the sample for more accurate model building. Also, consider differentiated privacy and federated learning for securely using sensitive data.
High complexity of integration with existing systems
Most companies work with the solution of an outdated technological stack, which is hard to integrate with modern AI algorithms. The difficulties are communication between individual system components, data transfer, and process synchronization.
Solutions
- Follow the modular approach: Developing artificial intelligence solutions as separate microservices allows for easy AI business integration into the existing ecosystem.
- Consider API usage and integration of middleware solutions: API and middleware integration allow for the continuous flow of data among systems.
- Migrate to the cloud: Using cloud platforms for data processing allows increasing scalability and flexibility of AI solutions.
High implementation costs
The development and training of AI models are expensive. Long projects that yield low returns in the initial stages of investment start to weigh heavily upon the company.
Solutions
ROI (return on investment) calculation before the project implementation: Expected outcome evaluation, cost projection, and economic viability assessment will avoid cost overruns.
Use of readymade solutions: The availability of solutions based on AutoML and other platforms enables you to cut costs considerably because there's no need to create your models.
Gradual implementation: AI solutions support step-by-step implementation processes. That means you can test the efficiency of a solution on a small scale before scaling.
Lack of expertise and technical skills
An engineer with deep AI and machine learning knowledge is still a rare resource. It is tough for companies without proper expertise to create effective processes of training models and their subsequent support.
Solutions
Investments in the training and retraining of specialists will help raise the level of professionalism. Then, cooperation with consulting companies and specialists in the field of AI can significantly accelerate the deployment process. In this respect, using tools to ease model setup and training saves the need for highly specialized expertise.
Explainability and trust issues in AI
It is critical for any business to know how and why a model makes a certain decision. Lack of transparency in AI predictions can destroy the trust of the users and regulators.
Solutions
Explainable AI (XAI) implementation: Explainable AI provides the means to facilitate better visualization and analysis of model decision-making processes.
Regular audits of algorithms for compliance: These help in model checking against expected results for problem identification.
Ethical standards and control of bias: Utilization of algorithms that reduce the risks of discrimination to the minimum and ensure decisions are objective.
Navigate AI challenges with Unwink.AI. Unlock smarter solutions today! Get in Touch
Identifying AI opportunities for business growth
A company that uses AI effectively always enjoys high competitive advantages because it increases business operations and can offer personalization to its customers. This transition from traditional business models toward expanded AI requires, besides technological change, strategic foresight.
At Unwink.AI, we help companies identify AI opportunities and implement them correctly. To maximize the impact of AI transformation, businesses must already possess these five essential attributes:
- Vision
- Skills
- Incentives
- Resources
- Action plan
If your company has the right ingredients, we’ll help turn them into a winning AI strategy.
How the lack of key attributes slows down change
If even one of these components is missing, a company may face difficulties on the path to AI implementation.
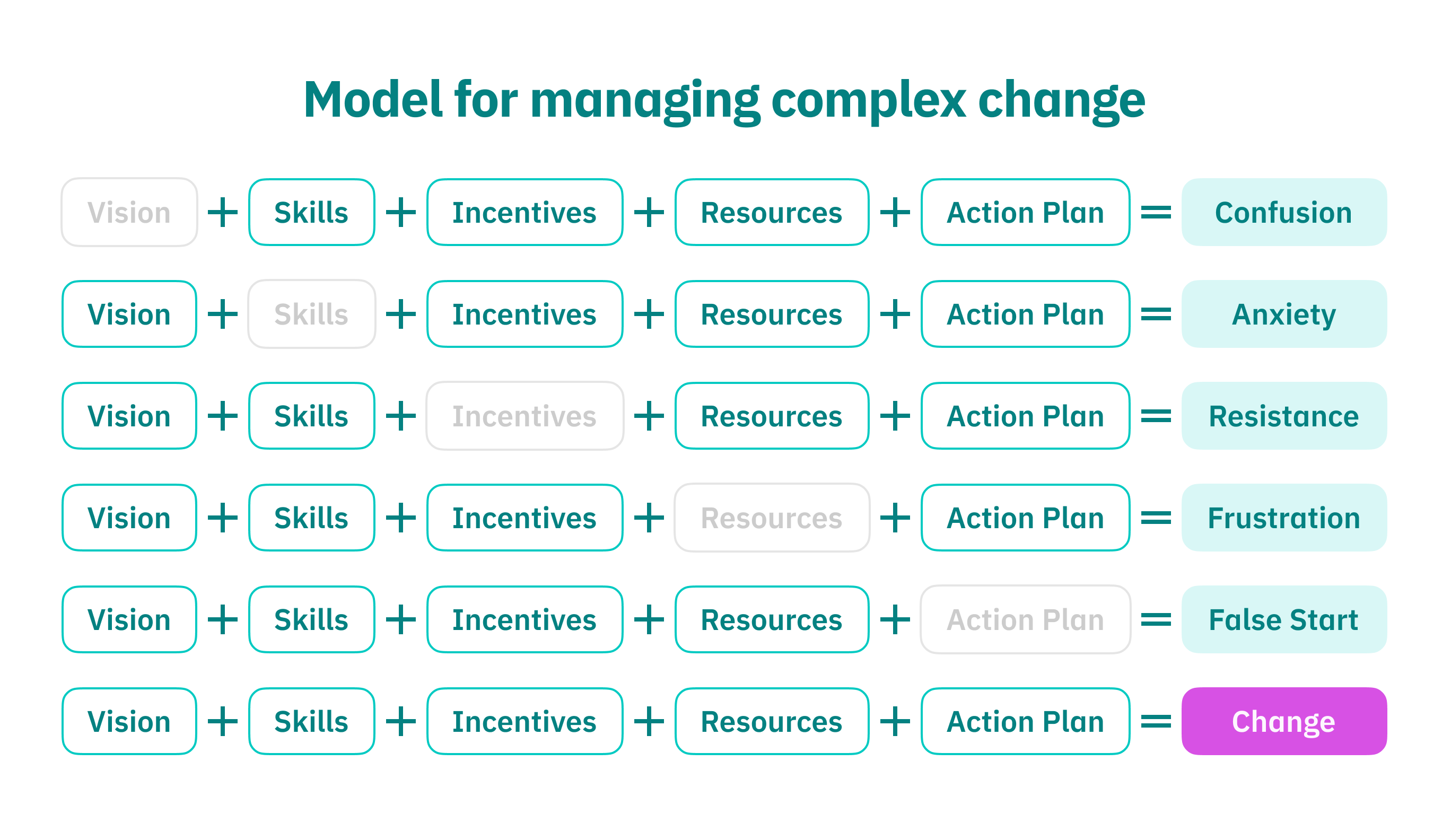
1. Lack of vision → confusion
Most companies without a clear AI implementation strategy tend to start experimentation without understanding what the end would look like. They, therefore, waste resources on PoCs that do not scale, or launch solutions that do not meet business goals.
At Unwink.AI, we analyze your business, identify areas where AI can have the greatest impact, and create an implementation roadmap.
2. Lack of skills → anxiety
Even the most ambitious plans often face a shortage of qualified specialists in companies. Without proper expertise, AI implementation can be marred by mistakes that slow down development.
Our data analytics platform provides automated solutions that minimize the need for highly specialized personnel. We also provide training for teams integrating AI.
3. Lack of incentives → resistance
Employees and managers who don't see a need for changes will resist them. Usually, AI is seen either as an immediate threat to jobs or as something too complicated to be worth the expenses.
We introduce real business use cases that clearly show how AI enhances workflows and does not substitute for people. It's also really easy to embed AI solutions without radical changes in business processes on our platform.

4. Lack of resources → frustration
Despite having vision, skills, and motivation, most companies can have limited financial and technical resources. That frustration follows when launching AI without support and finding the solutions does not yield the desired results.
Unwink.AI offers scalable AI solutions that fit your company's budget. Our models are optimized for cloud services to cut down on infrastructure costs.
5. Lack of a clear action plan → false starts
Companies with no gradual plan for integrating AI usually start projects without results. Companies either introduce several projects at once or stop after the first failures.
We at Unwink.AI work out an individual AI implementation plan according to needs and capabilities to achieve efficiency in a gradual transition from PoC to production.
To make AI integration work, an enterprise has to identify the capability of the technology and prepare its organization for change. Our data analytics platform develops solutions that overcome typical barriers along the way.
Conclusion
In 2025, successfully moving from PoC to AI implementation is a strategic process that requires a comprehensive approach. At this point, rigid infrastructure allows them to overcome all obstacles, permits continuous model enhancement, and follows best practices relating to security and ethics while using data. Those capable of adapting while envisioning future challenges will retain a competitive advantage and deliver further ways for growth and innovation.
Our data analytics platform enables companies to implement AI solutions smoothly, beating all technical and business obstacles from PoC to production. Contact us to discover how we can help your business make AI a competitive advantage!
FAQ
- How can companies measure the success of AI deployment?
Companies measure AI success by focusing on KPIs such as accuracy and efficiency gains, business impact metrics or cost reduction/revenue growth, and adoption rates. Continuous monitoring through A/B testing and feedback will ensure that AI remains effective and aligned with business goals.
- What are the most common reasons AI projects fail in production?
Poor data quality, undefined goals, scalability issues, and an inability to integrate are common reasons AI projects fail. Unrealistic expectations, regulatory hurdles, and lack of user adoption further hinder success. These can be overcome by continuous monitoring, collaboration across teams, etc.